Automatic Question Answering & Generation in News Archives
Abstract: The fields of automatic question answering, reading comprehension, and question generation have recently been rapidly advancing. Open-domain question answering, in particular, assumes automatically answering arbitrary user questions from a large document collection. The existing approaches are however designed to work on synchronic document collections such as Wikipedia, Web data, or short-term news corpora. We propose automatic question answering over temporal news collections which can contain millions of news articles that were published over several decades. Temporal aspects of both news articles and user questions form an additional challenge for this kind of question-answering task. We will first discuss a re-ranking approach for news articles which works by utilizing temporal information embedded in questions and in the underlying news archive, thus combining methods from Temporal Information Retrieval and Natural Language Processing. Next, we will discuss a dedicated solution for answering « When » type questions which require finding occurrence dates of events based on an underlying news archive. Finally, we will introduce ArchivalQA – a large-scale question answering dataset which has been automatically created from a two-decades-long news article collection, and which contains over 500k question-answer pairs. The dataset has been processed to remove temporally ambiguous questions and is designed for training question answering systems operating over long-term news archives.
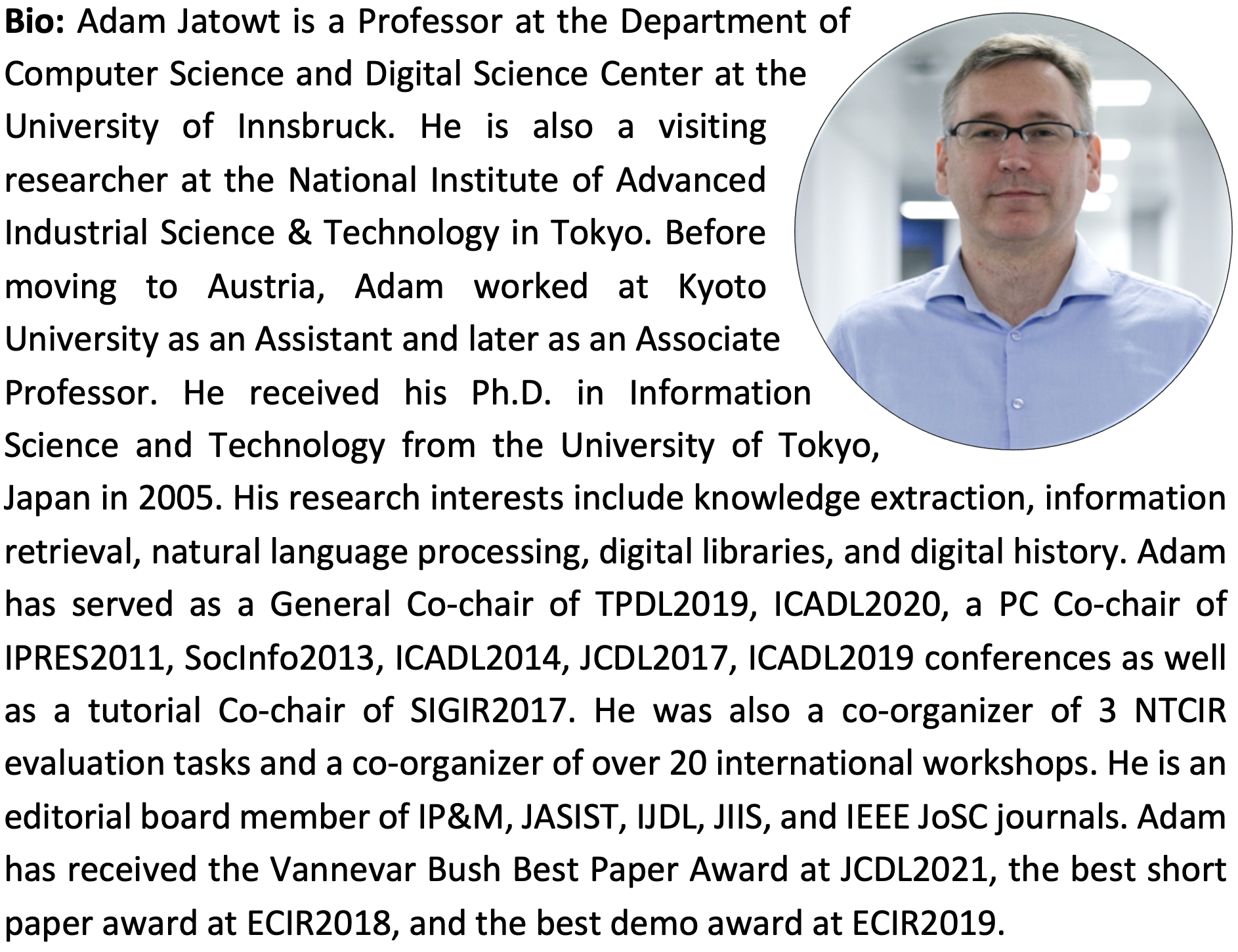
Slides
A PDF version of the slides is available here.
Location
Amphitheater of the building « Pôle Communication Multimédia Reseaux »
Date
Monday 23 May 2022, 8h45-9h30
For more details, please check the Scientific Program of DAS 2022 (https://das2022.univ-lr.fr/index.php/scientific-program/)